Sodankylän geofysiikan observatorio
Geofysiikan mittauksia ja tutkimusta vuodesta 1914
Sodankylän geofysiikan observatorio on Oulun yliopiston erillislaitos, joka sijaitsee revontuliovaalilla, 120 km Napapiirin pohjoispuolella.
Sodankylän geofysiikan observatorio on Oulun yliopiston erillislaitos, joka sijaitsee revontuliovaalilla, 120 km Napapiirin pohjoispuolella.
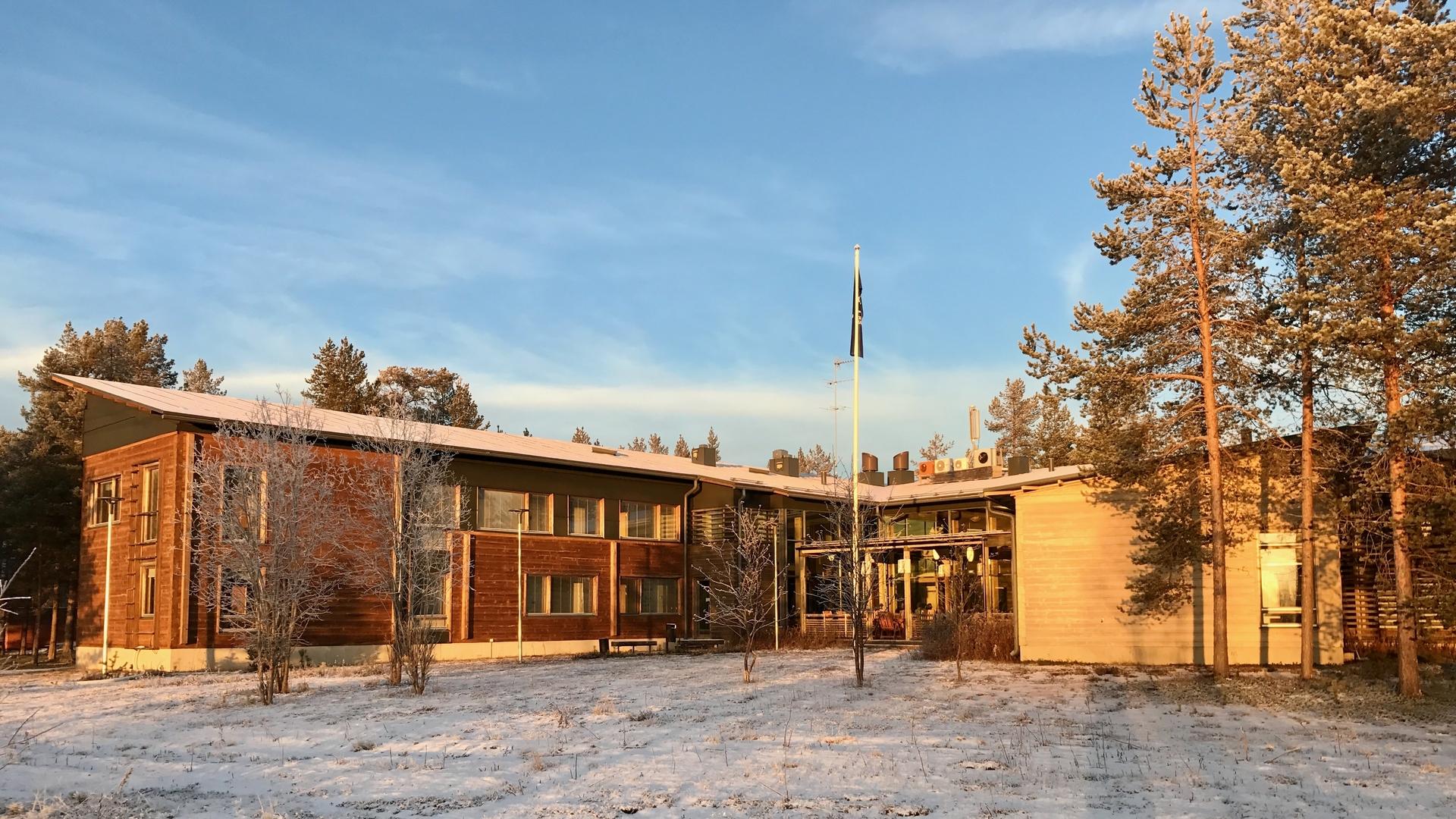
Suomalainen Tiedeakatemia perusti geofysiikan observatorion Sodankylään vuonna 1913 suorittamaan geofysikaalisia mittauksia ja tekemään mittausaineistoon perustuvaa tutkimustyötä. Observatoriotoiminta on siitä lähtien ollut jatkuvaa ja laajenevaa. Vuoden 1997 elokuun alusta lähtien Sodankylän geofysiikan observatorio on ollut Oulun yliopiston erillislaitos.
Pitkäaikaisiin mittauksiin ja mittausaineistojen tutkimustyöhön perustuva toiminta on vuosikymmenten saatossa kasvanut ja kehittynyt voimakkaasti. Nykyisellään observatorioalue tarjoaa maailman kattavinta omiin mittalaitteisiin ja mittauksiin perustuvaa Arktisen alueen seurantaa.