Biocenter Oulu
Biocenter Oulu maintains and continuously develops research infrastructure services for life sciences and biomedicine and it offers services with open access to support academic and other customers in their research and R&D projects. These services are part of the national distributed Biocenter Finland and the European EuroBioimaging, Infrafrontier and Instruct ESFRI research infrastructure networks.
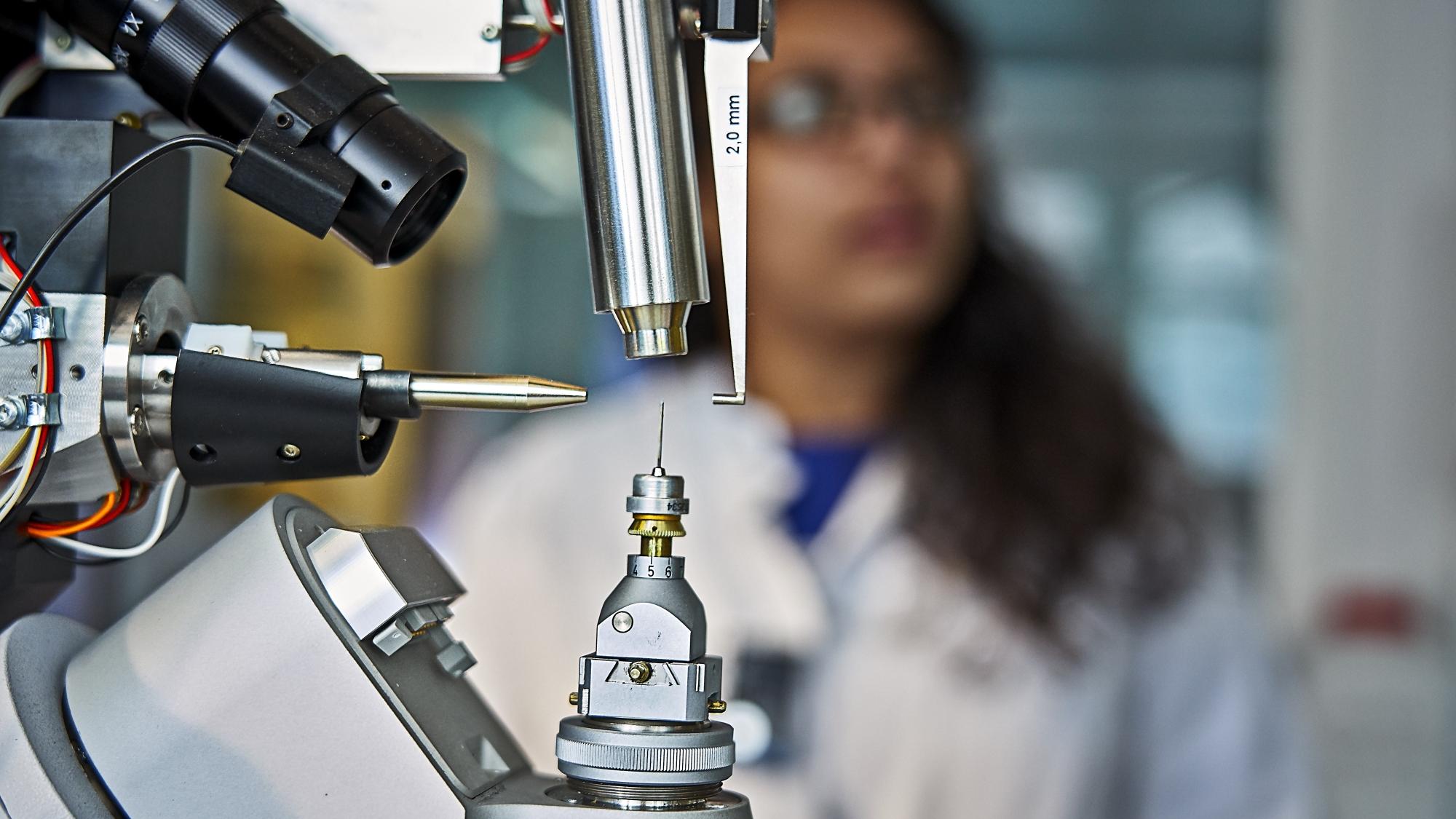
The University of Oulu’s strategy strengthens its role as a high-level international science and innovation university that contributes to solving global challenges through the selected focus areas of research. In the Human health and wellbeing research theme the strategic research projects are organized under Biocenter Oulu.
Kontinkangas campus infrastructure for biomedical research
Additional research infrastructure servides provided by the faculties
Biocenter Oulu Data Management Policy
Biocenter Oulu follows University of Oulu general data management policies and regulations and strives to ensure that all data produced by technology platforms will comply with FAIR principles.
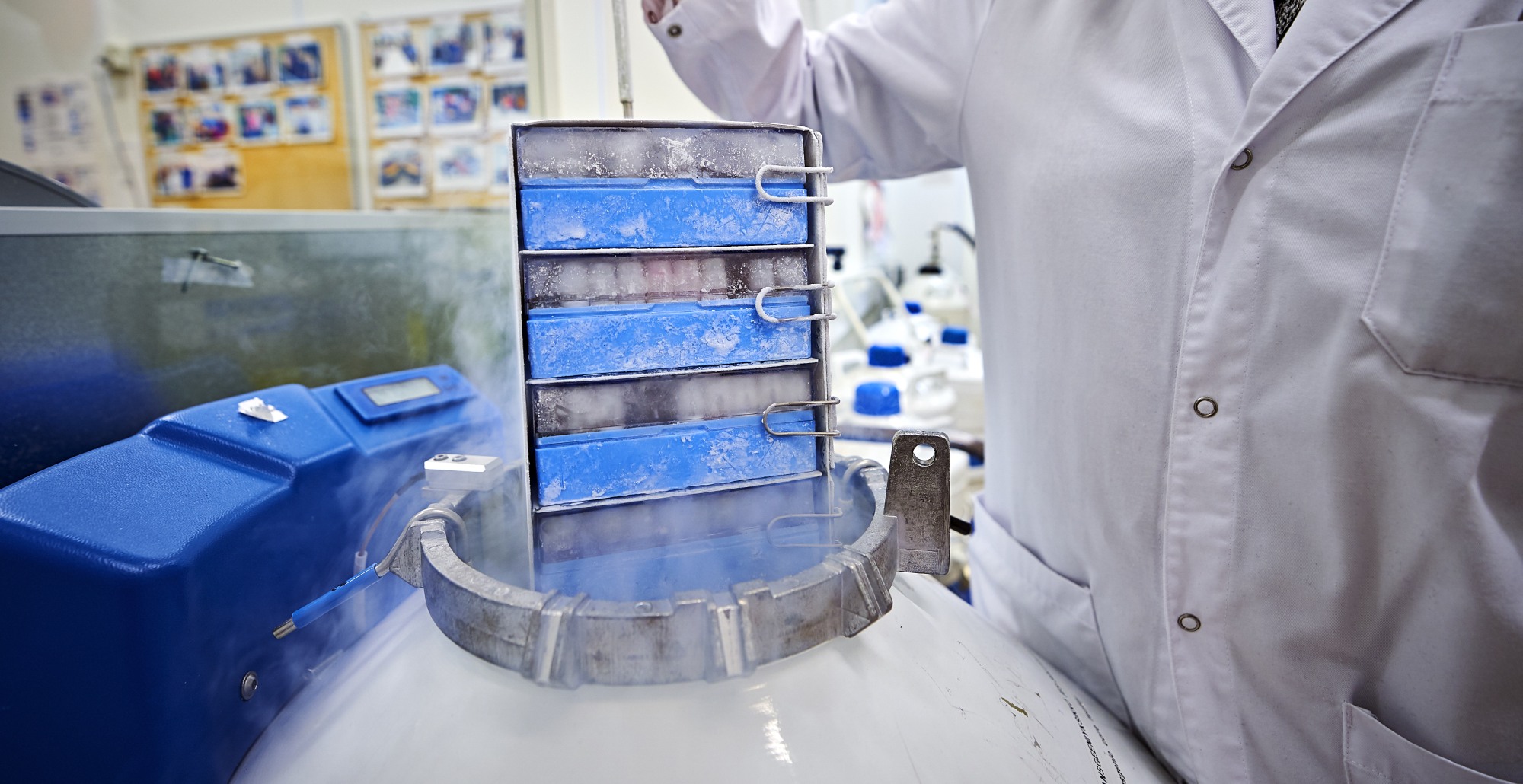
Health and Biosciences Doctoral Programme offers field specific doctoral training to its doctoral researchers by organizing courses and other activities and events.
Biocenter Oulu administrative office and majority of the core facilities are located at the Main Building of the Medical Campus, Aapistie 5A, which also houses some of the BCO research groups. The rest of the research groups are situated at the Faculty of Biochemistry and Molecular Medicine, Linnanmaa Campus and at the University Hospital.