Infotech
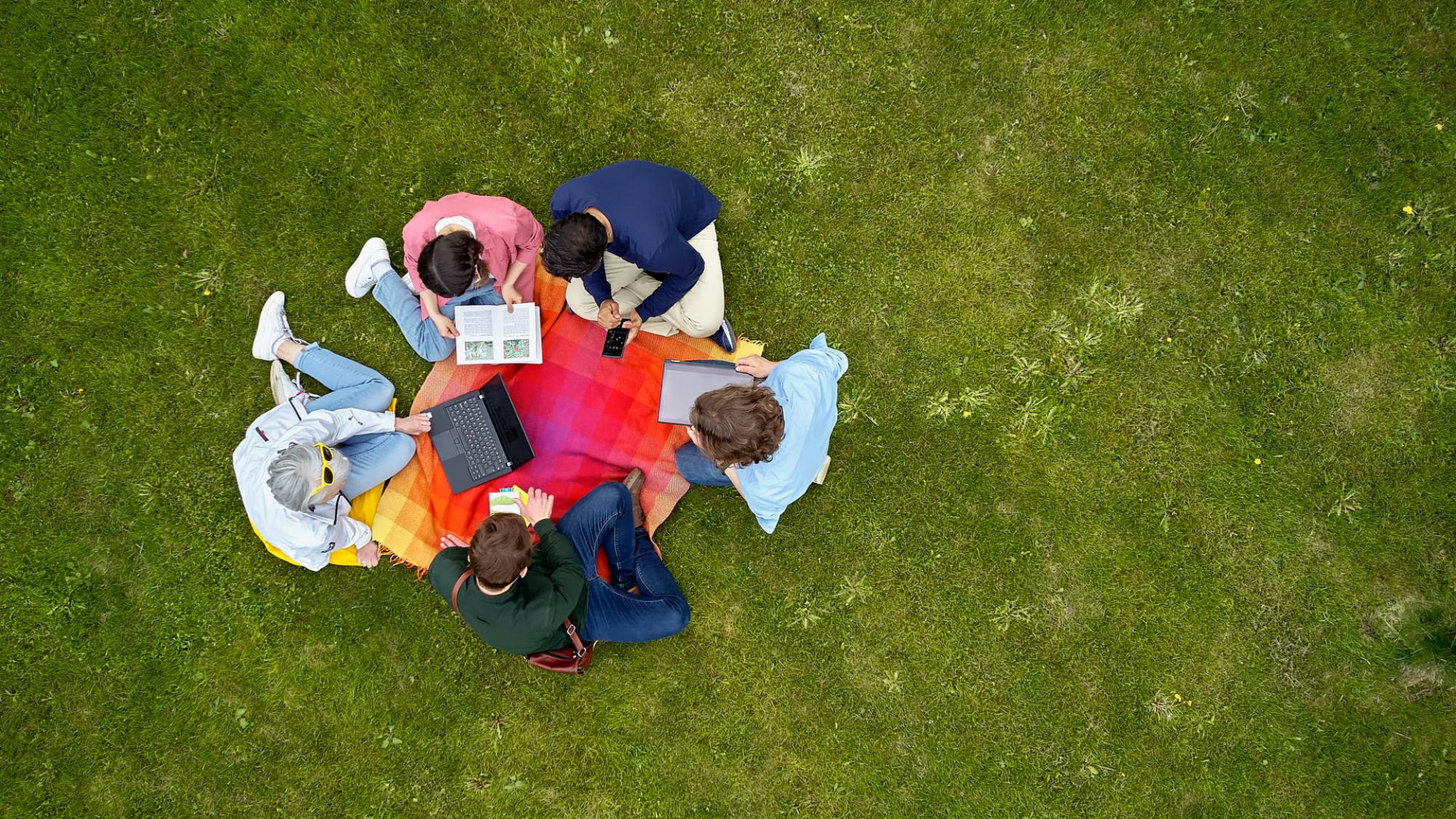
Infotech Oulu develops the University of Oulu's multidisciplinary and interdisciplinary research profile in close collaboration with the faculties. Infotech Oulu promotes, coordinates, and strengthens multidisciplinary research that addresses global challenges.
Infotech Oulu's research activities are supported by strategic university funding, which is allocated to 12-16 projects over four years, involving around 12 post-doctoral researchers and 17 doctoral researchers. These projects promote long-term research and doctoral training, especially targeting multidisciplinarity, to enhance the university's research profile and create new research openings. The projects cover a wide range of topics, including research in various fields of modern information technology for developing and validating, e.g., digital technologies, wireless sensors and networks, AI, machine vision, and electronics for various application areas.
A periodic report of research projects funded from 2018-2021 can be found here.
The Information Technology and Electrical Engineering (ITEE) Doctoral program offers field-specific doctoral training to its doctoral researchers. There are four doctoral degree programmes: Electrical Engineering, Communications Engineering, Computer Science and Engineering , and Information Processing Science.