Aleksei Tiulpin
PhD
Assistant Professor
Intelligent Medical Systems
Research Unit of Health Sciences and Technology
Faculty of Medicine
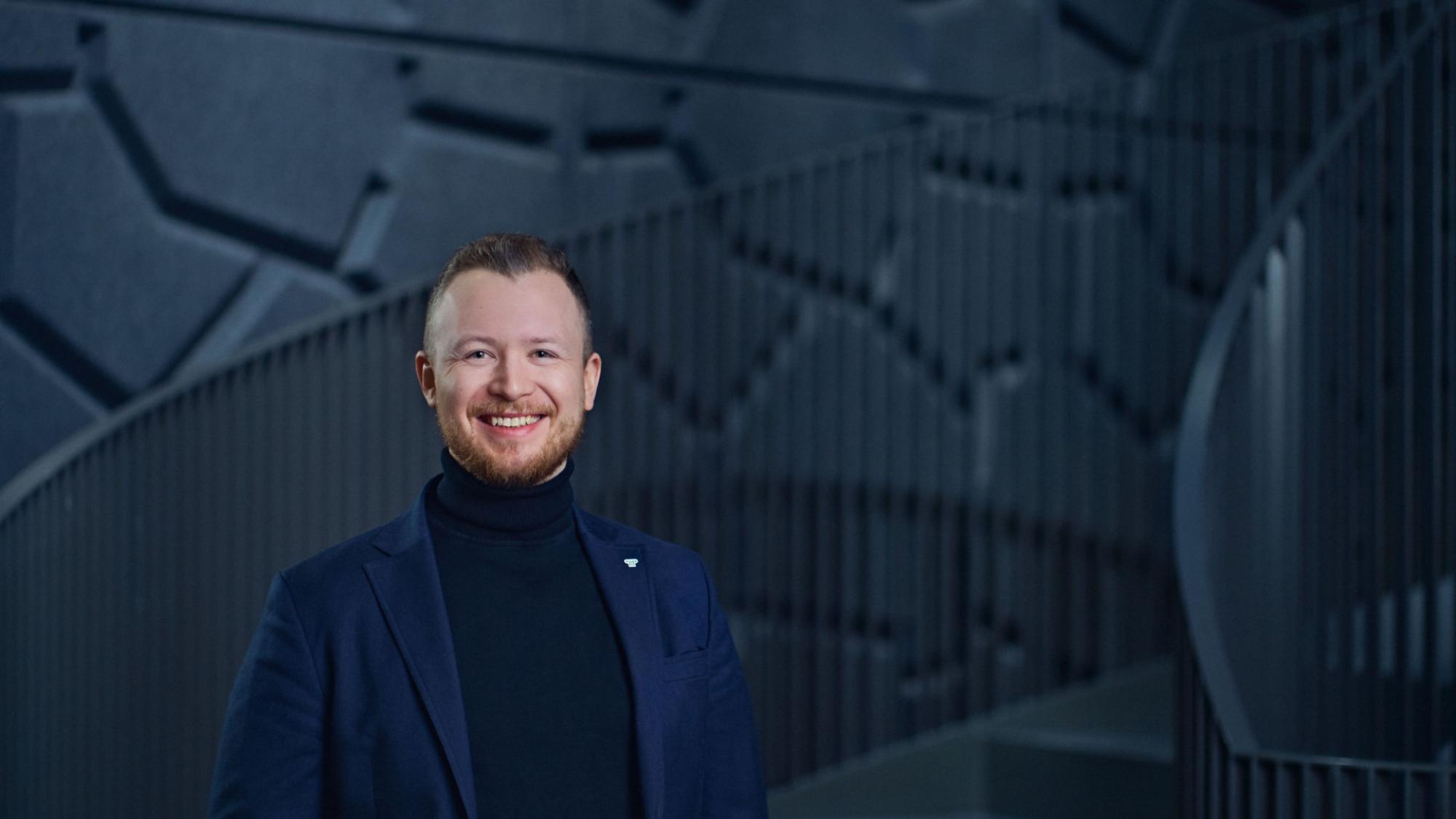
Bio-sketch
I am an Assistant Professor at the University of Oulu starting January 2022. I am also a member of the European Lab for Learning and Intelligent Systems (ELLIS). The focus of my research is on Intelligent Medical Systems, and I develop new machine learning methods for medical applications. Earlier, I was a post-doctoral fellow at the Finnish Center for Artificial Intelligence and Aalto University working with Prof. Samuel Kaski and Prof. Simo Särkkä. Before that, I was a post-doctoral fellow at KU Leuven, working with Prof. dr. Matthew Blaschko.
I earned my PhD during 2017-2020 (graduated with distinction) at the Faculty of Medicine, University of Oulu, Finland. My PhD thesis was nominated the best doctoral thesis of the year 2020. My advisors were Prof. Simo Saarakkala, PhD (primary), Dr. Jérôme Thevenot, PhD, and Prof. Esa Rahtu.
A title of Docent in Machine Learning for Medical Imaging was granted to me on 01.02.2022.
Current research
Generally, I am interested in all areas of Machine Learning, with a special focus on Deep Learning and Computer Vision. My main application area is medical imaging. My translational contributions are in the fields of on musculoskeletal disorders, breast cancer, and dementia.
My research agenda is to investigate how to build medical AI systems that
- Are multimodal
- Use as little labeled data as possible
- Predict how uncertain they are in their predictions
- Interact with humans and other AI systems
Research interests
- Machine Learning
- Medical Imaging
- Bayesian Deep Learning
- Human-in-the-loop Machine Learning
Researcher information
Research groups
Researcher profiles
Contact information