Andreas Hauptmann
PhD
Academy Research Fellow & Associate Professor
Computational Mathematics
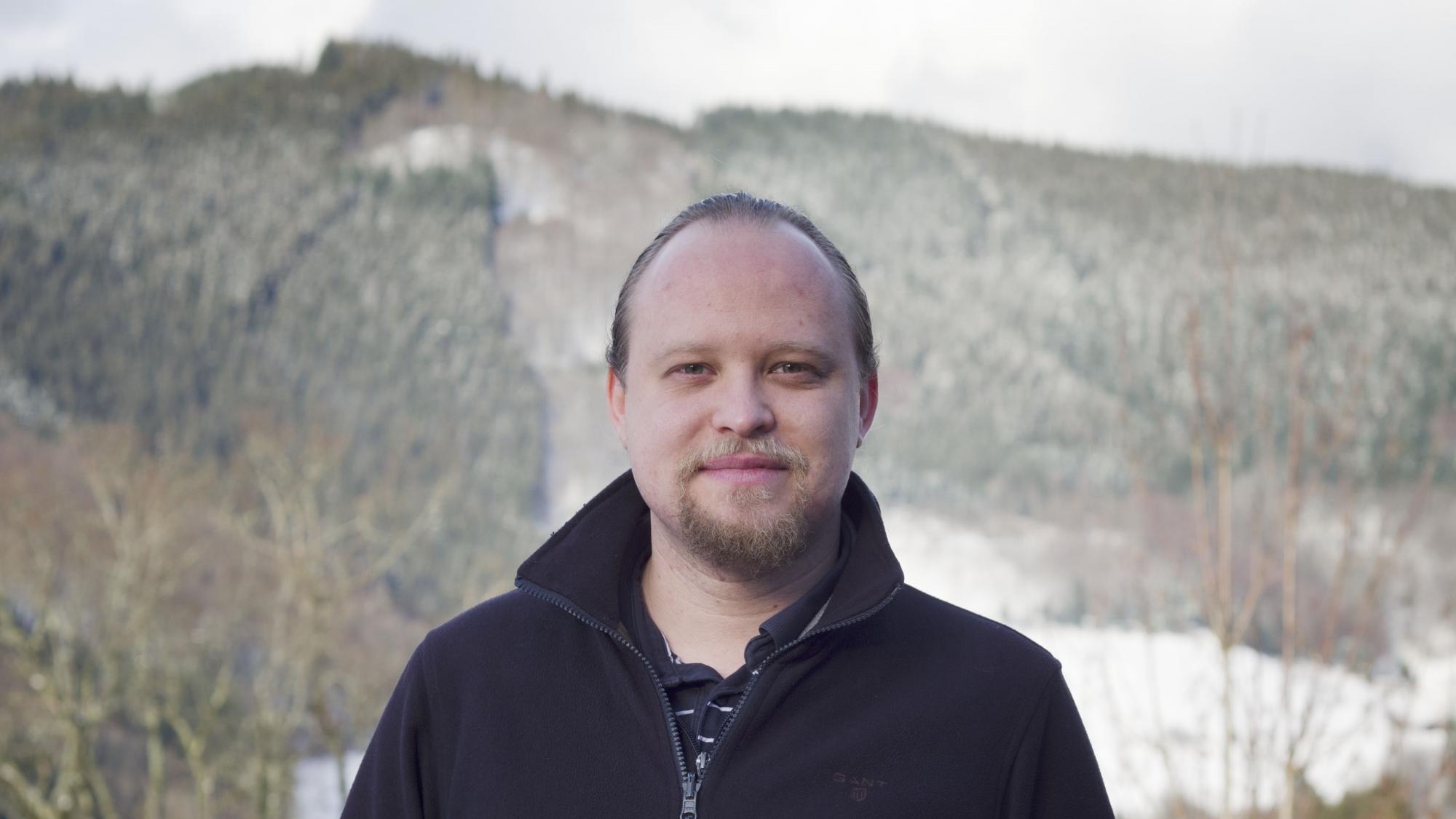
I am a computational mathematician interested in inverse problems and medical imaging, with expertise in tomographic reconstructions and image processing. My work is concentrated on combining analytical and data driven methods for industrial and medical applications.
In my Academy Research Fellow project, we will pioneer the necessary methodological advancements needed to enable Accurate Imaging with Sound and Light (AI-SOL) by combining artificial intelligence and mathematical theory in the novel field of learned image reconstruction to provide interpretable and reliable images for clinical practice.
My publications can be found on my personal homepage: www.hauptmann-research.net
Research interests
- Inverse Problems
- Computational Imaging
Researcher information
Researcher profiles
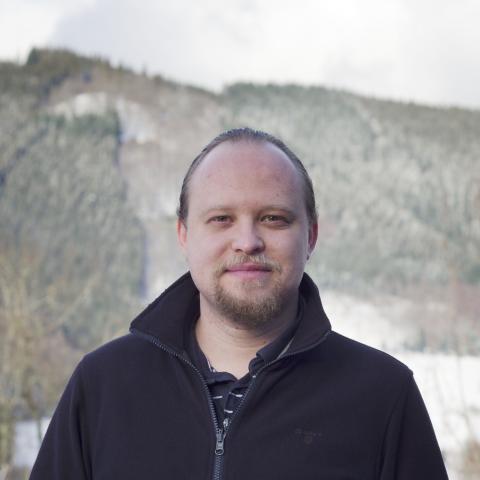
Contact information