Center for Machine Vision and Signal Analysis (CMVS)
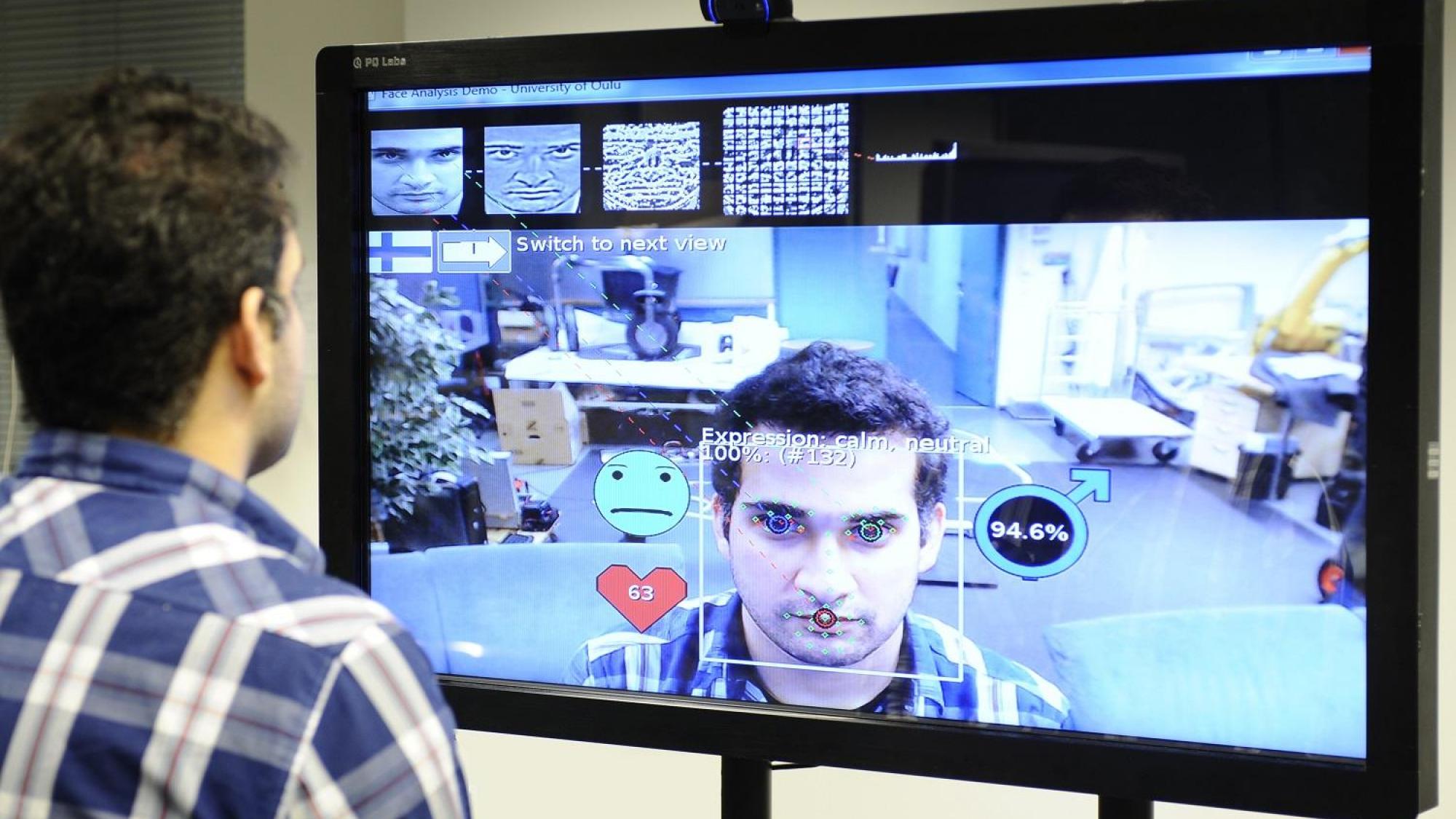
The Center for Machine Vision and Signal analysis has achieved ground-breaking research results in many areas of its activity, including texture analysis, facial image analysis, 3D computer vision, energy-efficient architectures for embedded systems, and biomedical engineering. Among the highlights of its research are the Local Binary Pattern (LBP) methodology, LBP-based face descriptors, and methods for geometric camera calibration, which all are highly-cited and widely used around the world. The areas of application for CMVS's current research include affective computing, perceptual interfaces for human-computer interaction, biometrics, augmented reality, and biosignal analysis.
Research groups
The Center for Machine Vision and Signal Analysis is composed of two research groups: Machine Vision Group and Physiological Signal Analysis Group.